Monte Carlo Analysis Software in Financial Decision-Making
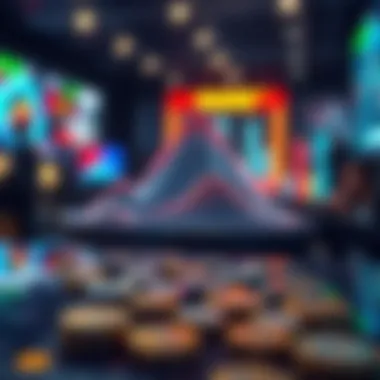
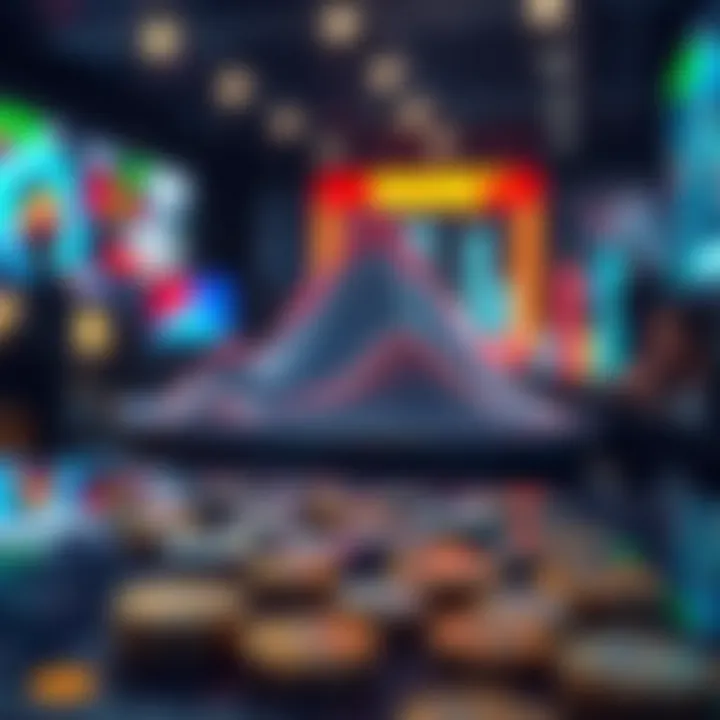
Intro
The financial landscape can often feel like a maze, with a multitude of variables influencing outcomes at every turn. In this intricate arena of investments, tools that aid in understanding risks and potential rewards become paramount. One such tool is Monte Carlo analysis software, which plays a crucial role in financial decision-making. As investors delve deeper into understanding their investments, the need for precise and data-backed decision-making becomes essential. Monte Carlo simulations provide the framework for evaluating risks by simulating various scenarios, thereby offering a view into the potential futures that might unfold based on current data and assumptions.
By engaging with this article, readers will unpack the essential principles of Monte Carlo simulations, explore diverse applications in multiple financial sectors, and gain insight into how this software can elevate their investment strategies. Investors, financial advisors, analysts, students, and entrepreneurs alike can benefit from a solid grounding in this software’s capabilities. From grasping foundational definitions to exploring expert insights, this deep dive intends to create a comprehensive guide that addresses both novice and seasoned practitioners in the field.
Moving forward, we will establish a baseline knowledge by defining key investment terms relevant to Monte Carlo analysis.
Foreword to Monte Carlo Analysis Software
In the ever-evolving world of finance, decision-making can often feel like walking a tightrope. With a plethora of factors influencing market outcomes, investors constantly seek reliable tools to guide their choices. That's where Monte Carlo analysis software comes into play. This software not only streamlines the complex calculations involved in predicting financial scenarios, but it also provides a robust framework for analyzing potential risks and returns. As we wander through this article, we will unpack how Monte Carlo simulations offer clarity amid uncertainty, and why they have become a cornerstone in financial modeling.
Definition and Purpose
Monte Carlo analysis can be likened to a crystal ball, albeit one grounded in mathematics rather than mysticism. At its core, this technique utilizes random sampling to understand and predict the range of possible outcomes in any given scenario. For financial analysts, the purpose is clear: to simulate a multitude of market conditions and their potential impacts on investments, portfolios, or any financial undertaking.
In essence, these simulations allow investors to move beyond straightforward predictions and delve into probabilities. By generating thousands, if not millions, of possible scenarios based on historical data and certain assumptions, analysts can attain a broader perspective on potential risks. The ultimate goal is to facilitate informed decision-making in investment strategy, enabling stakeholders to weigh the possible rewards against the risks.
Historical Development
Diving into the backstory of Monte Carlo analysis is like peeling an onion—layer upon layer reveals its growth through time. The technique traces its origins back to the early 20th century, with roots embedded deeply within the fields of physics and gambling (hence the term ‘Monte Carlo’). The method truly took flight during World War II when scientists were wrestling with uncertainties related to atomic research. By the 1970s, financial analysts began adapting these simulations to assess overall investment risks, marking a significant evolution of the technique.
Fast forward to today, and we see Monte Carlo simulation software taking on myriad forms and capabilities, from simple calculators to highly sophisticated platforms equipped with robust graphical interfaces. This progression has made Monte Carlo methods more accessible, enabling a wider spectrum of investors to harness their power.
Relevance in Investment Strategies
Thinking strategically is crucial for any investor. The relevance of Monte Carlo analysis in investment strategies cannot be overstated. In an age where an abundance of data is available, the ability to visualize various outcomes becomes paramount. Utilizing this software, investors can experiment with different scenarios—what if interest rates rise? Or, what if market volatility spikes?
Key benefits include:
- Risk Assessment: By simulating countless variables, one can identify high-risk scenarios that might otherwise go unnoticed.
- Portfolio Optimization: Investors can refine their portfolios, testing how different assets might perform under various conditions.
- Enhanced Understanding: It educates users about the probabilities associated with different financial outcomes, deepening their understanding of market nuances.
In summary, Monte Carlo analysis software provides invaluable insights that transform traditional investment methods into more sophisticated, data-driven strategies. As we continue this exploration, we will delve deeper into the core principles and practical applications, emphasizing how this powerful tool can significantly sharpen financial decision-making.
Core Principles of Monte Carlo Simulations
The essence of Monte Carlo simulations rests on a few foundational principles that are crucial for understanding how this methodology operates within financial contexts. These principles not only undergird the statistical soundness of simulations but also elucidate their practical applications in investment decision-making. By grasping these core tenets, financial analysts and investors can leverage the full potential of this powerful analytical tool.
Random Sampling Techniques
At the heart of a Monte Carlo simulation is the use of random sampling techniques. This approach fundamentally hinges on the idea of generating a vast array of possible outcomes derived from known probability distributions. For instance, imagine you are evaluating the future return distribution of a stock based on historical performance data. By employing random sampling methods, such as uniform or Gaussian distributions, you can create numerous possible scenarios by generating random values that correspond to those distributions.
The crucial benefit of random sampling lies in its ability to mimic real-world uncertainties and variabilities, allowing investors to simulate a diverse range of possible market conditions. This process can uncover potential risks and returns that traditional deterministic models may gloss over. Moreover, it incorporates the inherent randomness of financial markets into the analysis, reflecting the unpredictable nature of investments.
Probability Distributions
Understanding probability distributions is vital when utilizing Monte Carlo simulations. These distributions provide the framework within which the random variables operate. Commonly used distributions in finance include the normal, log-normal, and exponential distributions.
For a tangible example, when modeling stock prices, the log-normal distribution is often preferred. This preference arises because stock prices cannot drop below zero, and the log-normal model accommodates this aspect while reflecting the growth potential of asset prices.
Through these distributions, financial professionals can gauge the likelihood of different outcomes based on historical data or assumed conditions. It establishes a foundation that allows negotiations of risks and rewards clearer, helping in making informed decisions that go beyond mere guesswork. The correct choice of distribution can significantly influence the interpretation of the results, making it paramount for users to be not just procedural, but discerning in their selections.
Statistical Analysis and Outcomes
The final piece of the Monte Carlo puzzle involves statistical analysis of the outcomes generated through random sampling and distributions. Each simulation run creates a multitude of potential results, which can be then subjected to statistical scrutiny to extract valuable insights.
"Statistical analysis of Monte Carlo outputs allows investors to visualize risks and project returns, making the abstract concrete."
Key metrics derived through this process might include the mean, variance, standard deviation, and even percentiles, which collectively paint a comprehensive picture of potential investment performance. For instance, by analyzing the results, an investor might discover that while a particular strategy exhibits a high mean return, it also carries significant risk as evidenced by high volatility.
This statistical analysis facilitates effective risk management and can empower financial advisors to guide their clients toward strategies that are more aligned with their risk tolerance. Refined understanding leads to more robust decision-making frameworks, enabling the optimization of portfolios tailored to individual objectives.
Ultimately, the blend of randomness, appropriate distributions, and thorough statistical scrutiny forms the backbone of successful Monte Carlo simulations, providing a nuanced understanding of potential investment landscapes.
Applications of Monte Carlo Analysis in Finance
In the realm of financial decision-making, the applications of Monte Carlo analysis stand out as indispensable tools. These applications extend far beyond mere number crunching; they involve the intricate interplay of probabilities and diverse financial scenarios which help investors and analysts gauge potential risks and rewards. Employing Monte Carlo simulations enables one to visualize a spectrum of outcomes, offering insight that is often overlooked by traditional analytical methods. Understanding these applications is crucial for anyone navigating the complexities of finance.
Portfolio Management
When it comes to portfolio management, Monte Carlo analysis aids in constructing a diversified portfolio that aligns with risk tolerance and financial objectives. By simulating thousands of potential future returns for investments, investors can observe the behavior of a portfolio under various market conditions. This richer understanding of potential performance helps in making informed decisions.
For instance, if an investor is considering a mix of stocks and bonds, they can simulate how this particular combination might perform in a bearish market versus a bullish one. Each simulation provides data on expected returns and risk levels, guiding investors to adjust their portfolios accordingly. When doing so, it's essential to keep in mind the historic correlations and volatilities between different assets, as these factors heavily influence the simulations.
"Monte Carlo simulations can help unveil the layers of portfolio dynamics, making the invisible visible."
Risk Assessment and Management
Risk assessment is another area where Monte Carlo analysis proves invaluable. It allows analysts to quantify uncertainty and assess the probabilities of different adverse financial events. By creating simulations that reflect potential economic downturns or market fluctuations, firms can prepare better risk management strategies.
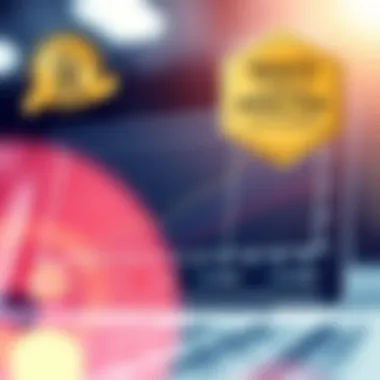
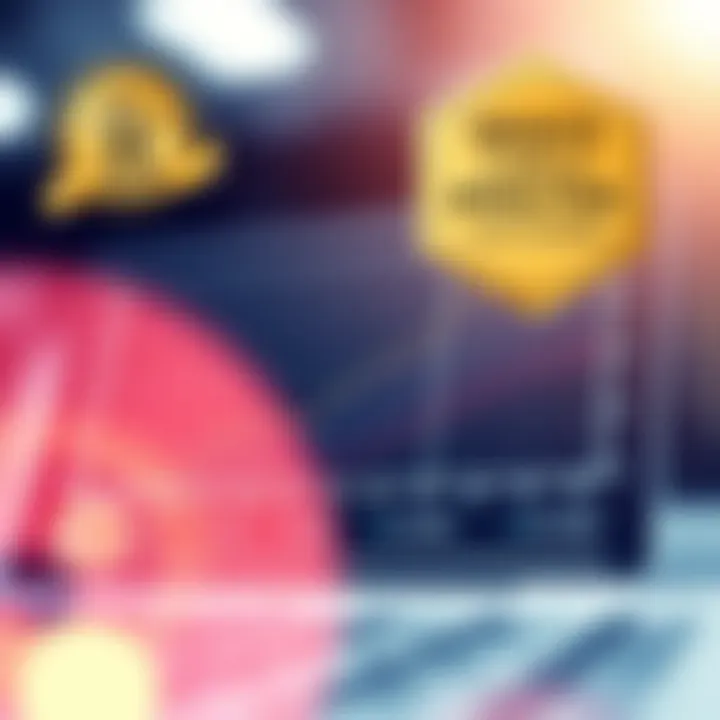
For example, banks and financial institutions often apply Monte Carlo simulations to evaluate their capital adequacy under various stress scenarios. This proactive approach fosters resilience and equips organizations with information essential to withstand market shocks. Additionally, the capacity to model the impact of changing interest rates, credit defaults, and liquidity constraints plays a vital role in refining risk management protocols.
Option Pricing Models
In the field of derivatives, particularly options trading, Monte Carlo methods offer sophisticated solutions for pricing and risk assessment. Unlike traditional analytical models, Monte Carlo simulations can accommodate complex options with complicated payoffs and path-dependent features.
For instance, consider the pricing of Asian options, which depend on the average price of the underlying asset over a certain period. Monte Carlo methods enable traders to simulate various price paths that the asset may take and, in turn, calculate the option pricing with greater accuracy. This flexibility not only enhances pricing precision but also helps in managing the associated risks by allowing traders to visualize potential payoffs in various scenarios.
Project Valuation
Last but certainly not least, Monte Carlo analysis plays a critical role in project valuation, particularly when assessing projects with uncertain cash flows. By simulating a range of potential revenue outcomes over different time horizons, decision-makers glean insights into the viability of a project before committing financial resources.
For instance, when evaluating real estate projects, investors can use Monte Carlo simulations to account for variations in property values based on market conditions and economic factors. By leveraging this approach, stakeholders can better assess the expected return on investment and the risks involved, enabling them to make choices grounded in a broader understanding of potential financial outcomes.
In summary, the applications of Monte Carlo analysis in finance not only illuminate the path for effective decision-making but also equip financial professionals with the analytical rigor needed to understand and respond to the complexities of the market.
Comparative Analysis of Monte Carlo Software Tools
In the world of finance, choosing the right tools can be a make-or-break aspect of decision-making. The comparative analysis of Monte Carlo software tools plays a crucial role in highlighting the strengths, weaknesses, and unique features of various software available in the marketplace. As investment professionals continuously strive for precision in risk assessment and financial forecasting, understanding these tools becomes pivotal.
This analysis isn’t merely an academic exercise; it has practical implications that affect both strategy and outcomes. By scrutinizing available software, investors can align their objectives with the right solutions—ensuring that they wield high-performance tools that enhance their capabilities.
Criteria for Evaluation
When evaluating Monte Carlo software, several criteria stand out. Investors and analysts typically consider factors such as:
- Ease of Use: Software should be user-friendly; complexity can lead to errors in large data sets.
- Flexibility: The capacity to customize simulations based on specific investment scenarios is vital.
- Integration Capabilities: Good software should seamlessly integrate with existing systems and databases.
- Output Clarity: The presentation of simulation results plays an essential role in effective interpretation and decision-making.
- Cost Efficiency: Understanding the price versus the feature set helps gauge overall value.
These elements aid in discerning which software packages align with particular investment strategies and risk profiles.
Three Leading Monte Carlo Software Packages
Not all Monte Carlo tools are created equal; each has its unique offerings. Among the top contenders are:
- Crystal Ball: Known for its advanced spreadsheet integration and a wide array of statistical analysis tools. Crystal Ball supports risk analysis and forecasting in a familiar Excel environment, attracting many financial professionals.
- @RISK: A well-regarded software that excels in its user-friendly interface. It integrates well with Microsoft Excel and provides extensive scenario analysis, which is crucial for portfolio risk assessment.
- Risk Simulator: This software is often lauded for its robust simulation capabilities and user-friendly dashboard. It allows for extensive input variety, making it ideal for highly technical analysis.
These software packages have been vetted and utilized in diverse financial landscapes, giving them credibility and reliability.
Cost-Benefit Analysis of Features
Understanding the cost-benefit balance is essential for any financial decision. Here, we take a closer look at features offered by these software packages:
- Crystal Ball: Offers advanced analytics but requires a significant financial investment. Its learning curve may be steep for novices, but seasoned professionals reap vast benefits.
- @RISK: Generally more affordable; it provides most essential features for typical use cases without breaking the bank. However, users may find some advanced functionalities lacking.
- Risk Simulator: Priced competitively and suitable for mid-level applications, it provides excellent basic functionalities while potentially leaving advanced users wanting in specific areas.
By carefully weighing these factors, investors can make informed decisions that best fit their financial strategies and budgetary constraints.
A comprehensive understanding of these tools empowers financial professionals to extract the utmost value from Monte Carlo simulations, leading to better-informed investment strategies.
Limitations of Monte Carlo Analysis Software
While Monte Carlo analysis software can be an invaluable tool in financial decision-making, it doesn’t come without its shortcomings. Acknowledging these limitations is crucial for investors, financial advisors, and analysts who seek to fully harness its potential while being mindful of its boundaries. Understanding these factors can lead to more prudent investment strategies and risk management approaches.
Reliance on Assumptions
One of the central tenets of Monte Carlo simulations is that they are heavily dependent on input assumptions. These assumptions include the choice of probability distributions, expected returns, and volatility. If these inputs are not grounded in robust data and realistic scenarios, the results can be misleading. For instance, if an investor presumes that historical returns will continue in the same manner moving forward, they might find themselves on a slippery slope when market dynamics shift.
Moreover, some investors might favor overly optimistic scenarios leading to inflated risk assessments. This becomes problematic when crafting portfolios or making decisions based purely on these simulations. To mitigate these issues, it’s essential to regularly revisit the input data and assumptions used, particularly in periods of market upheaval. As the saying goes, "don’t put all your eggs in one basket"; ensuring a variety of scenarios while testing your assumptions can greatly enhance the reliability of the analysis.
Computational Complexity
The computational demands of Monte Carlo simulations can pose significant challenges, particularly for firms lacking the necessary technological infrastructure. Complex models require substantial computing power, and running extensive simulations could lead to increased operational costs. Some organizations might be caught off guard by how much time and resources are consumed by such calculations, especially if the simulations are not efficiently designed.
In a practical situation, a small hedge fund trying to model intricate derivative options might find themselves hamstrung by computational limitations. Each run of the simulation may consume considerable time and resources, leading to a frustrating bottleneck in decision-making processes.
To overcome these obstacles, it's prudent for organizations to invest in suitable software packages and hardware solutions, or potentially look into outsourcing computational needs to cloud providers who specialize in high-performance computing. This step can not only optimize the speed of analysis but also offer scalability that many firms require.
Potential for Misinterpretation
As with any analytical tool, results derived from Monte Carlo simulations can be misinterpreted. The presentation of outcomes, statistical measures, and sensitivity analyses can lend themselves to distortion. For example, the probabilistic outputs may be construed by some as guarantees rather than estimates. This misconception can lead investors down the path of false confidence, especially if they misconstrue the ranges for expected returns.
It's particularly important for stakeholders who are not deeply versed in statistical analysis to recognize the difference between a confident prediction and a probabilistic outcome.
"Statistically significant does not translate to materially significant."
In other words, a result might indicate a high probability of a certain outcome, but the implications of that outcome may not be as impactful in the real market context. Regular training sessions or workshops focused on interpreting such analyses can greatly empower teams to make informed decisions with a clearer understanding of the various outputs presented.
In summary, while Monte Carlo analysis software is a powerful instrument in the financial toolbox, recognizing its limitations will lead to more informed and effective usage. Investors, advisors, and analysts alike must approach these models with a critical mindset and ensure constant vigilance in validating their assumptions, understanding computational needs, and interpreting the results comprehensively.
Challenges in Implementing Monte Carlo Simulations
When it comes to diving into the nitty-gritty of Monte Carlo simulations, understanding the hurdles is just as crucial as grasping the principles behind the methodology. This section underscores the significance of the various challenges faced by investors and financial analysts when implementing these sophisticated analytical tools. Facing these challenges head-on ensures that the benefits of Monte Carlo simulations are maximized while mitigating potential pitfalls.
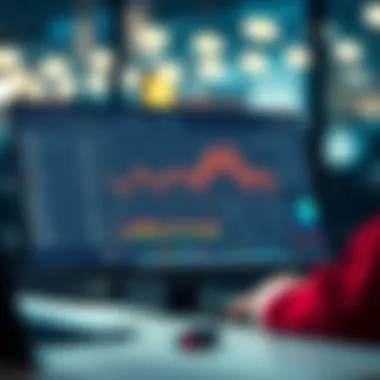
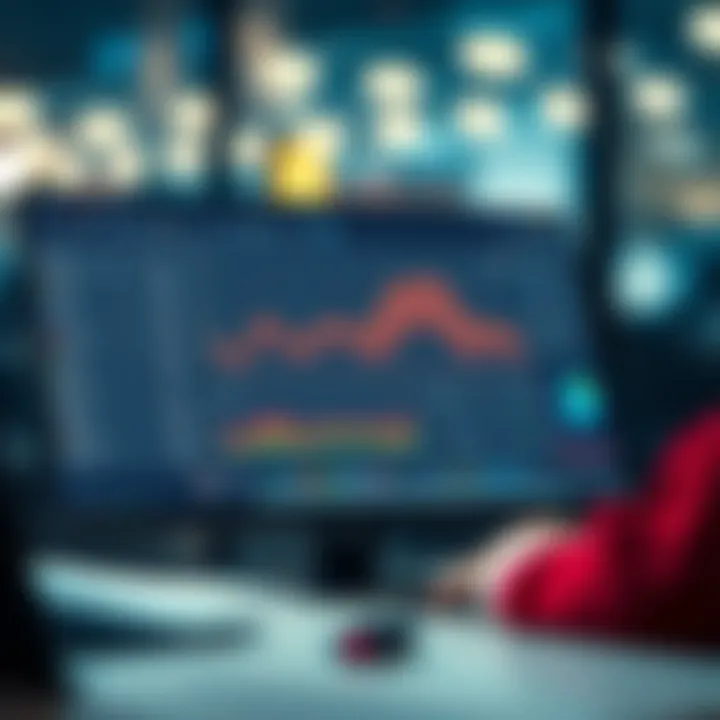
Data Quality and Availability
In the world of finance, garbage in means garbage out. The strength of any Monte Carlo analysis hinges on the quality of the data fed into the simulations. Investors need accurate, timely, and relevant data to generate reliable projections. However, obtaining such data can be a challenging endeavor. Often, financial datasets are laden with inconsistencies, gaps, and, sometimes, irrelevant information. For instance, mining data from disparate sources may lead to discrepancies, which could muddy the waters of analysis.
Moreover, there's the issue of availability. Some optimal datasets are not readily accessible or come with high costs. Investors and analysts might find themselves staring at a black hole when seeking comprehensive datasets that can enhance their simulation outputs. Lacking this data not only skews results but can also impede decision-making processes.
Without high-quality and readily available data, the accuracy of Monte Carlo simulations cannot be ensured.
To counter these challenges, it's imperative to establish robust data governance frameworks that prioritize data integrity and consistency. Organizations would benefit greatly from investing in reputable data sources and technologies that ease data acquisition and validation.
Integration with Existing Systems
Another noteworthy challenge is the integration of Monte Carlo software with existing financial systems. Often, companies utilize a myriad of software solutions—from trade execution systems to portfolio management tools. Ensuring these disparate systems work together synergistically can be quite the task.
Conflicts between software architectures may arise, leading to potential errors in data transfer or, worse, lost data. For example, a Monte Carlo simulation tool requiring specific input formats might confront hurdles when importing data from an existing financial database that uses a different structure. This disconnect can result in wasted time and resources, going against the efficiency that such simulations aim to gain.
A common strategy to address these integration issues is to opt for software solutions that offer open APIs. These allow for smoother communication between systems, enabling real-time data synchronization and enhancing the overall functionality of the tools in use.
User Expertise Requirements
Lastly, we can't overlook the elephant in the room: user expertise. Monte Carlo simulations, while powerful, are not exactly a walk in the park. Users need a firm understanding of statistics, finance, and the specific software being used. This means that financial models can’t be left solely to wishful thinking; they need to be informed by users who know their onions.
Many firms face a shortage of qualified personnel who can navigate these complex instruments effectively. This shortage can have ripple effects, where user errors can lead to misinterpretations, potentially resulting in poor investment decisions.
Organizations should think about training programs to enhance expertise among their teams. Providing educational resources and ongoing support can diminish the steep learning curve associated with Monte Carlo simulations. Investing in human capital is just as crucial as the technology itself.
In summary, while Monte Carlo simulations offer profound insights into financial decision-making, overcoming the challenges of data quality, system integration, and user expertise is paramount. Thus, understanding these hurdles becomes an important aspect of harnessing their full potential.
Advancements in Monte Carlo Analysis Technology
In recent times, the world of Monte Carlo analysis software has witnessed significant advancements, fundamentally changing how investors, analysts, and financial advisors approach their decision-making processes. These changes are not just cosmetic but are rooted in deeper technological updates that enhance accuracy, usability, and integration. Such improvements make this analytical tool more powerful and relevant in today's fast-paced financial environment.
Incorporation of Machine Learning
The integration of machine learning into Monte Carlo simulations marks a critical turning point for analytical processes. Whereas traditional simulations rely heavily on static data and predefined parameters, machine learning enables the software to adapt and learn from historical data patterns. This adaptability enhances the accuracy of predictions by allowing the program to update its algorithms based on new information, thereby refining the forecasts continuously.
For example, an investor might use Monte Carlo software that integrates machine learning to evaluate a new stock. As market conditions change, the software adjusts its parameter values allowing for real-time updates in risk analysis. This provides investors with a dynamic view of their portfolios, making them better equipped to manage potential downturns and recognize emerging opportunities.
Cloud-Based Solutions and Accessibility
The shift to cloud-based solutions has democratized access to Monte Carlo analysis software. Previously, owning high-end simulation software meant hefty upfront costs and maintaining complex hardware systems. Now, cloud platforms allow users to access robust Monte Carlo simulations via a subscription model. This has three main benefits:
- Lower Costs: Users do not need to invest in expensive infrastructure.
- Scalability: Resources can be adjusted based on demand, allowing users to scale their analytical needs as their portfolios or projects grow.
- Collaboration: Cloud environments foster easier collaboration among teams, allowing for multiple users to work on simulations concurrently. This is especially beneficial for advisory firms where teamwork is essential for thorough analyses.
User Interface Improvements
In a world where technology changes at breakneck speed, the importance of a well-designed user interface in Monte Carlo analysis software cannot be overstated. User interfaces are becoming more intuitive, catering to both novices and seasoned experts alike. Improved UI can significantly reduce the learning curve associated with these complex analytical tools.
Modern software now features:
- Drag-and-drop functionalities for inputting variables,
- Visual dashboards that offer real-time data visualization, and
- Step-by-step guides that simplify complex tasks.
These enhancements do more than just improve aesthetics; they enhance understanding and encourage wider adoption. An enhanced UI allows better decision-making as it facilitates easier interpretation of results and faster responses to market changes.
"Technology is best when it brings people together."
In summary, advancements in Monte Carlo analysis technology encapsulate the essence of current trends aimed at improving usability, accuracy, and accessibility for investors and analysts alike. As these transformations unfold, they pave the way for smarter financial decision-making, empowering users to leverage Monte Carlo simulations in ways that were once out of reach.
Best Practices for Utilizing Monte Carlo Analysis Software
When it comes to financial decision-making, using Monte Carlo analysis software is not just about crunching numbers; it's about making informed choices that can significantly impact outcomes. Following best practices can greatly enhance the effectiveness of these simulations. Well-crafted parameters and an ongoing commitment to diligence can lead to better conclusions and more robust strategies. It is crucial that investors and analysts alike recognize the key elements that play a role in refining their approach towards Monte Carlo methods.
Setting Realistic Parameters
Setting the right parameters is like laying down the foundation of a sturdy building. If the base is shaky, the entire structure might collapse. For Monte Carlo simulations, parameters refer to the variables set to represent the financial scenario being analyzed. They should be grounded in reality. Speculating unrealistic return rates or overly optimistic assumptions can lead to misleading outputs.
Consider the following points when setting parameters:
- Historical Data: Utilize relevant historical data to inform your parameters. This helps ground your input in real-world performance over various market cycles.
- Volatility Estimates: Accurate measurements of volatility from past performance can guide settings for the expected range of returns.
- Market Conditions: Be attentive to current market conditions. When setting parameters, it's essential to understand that volatility can shift and expectations should adapt correspondingly.
Conducting Sensitivity Analysis
Sensitivity analysis examines how changes in input variables affect the outputs of a model. It’s critical for understanding risks involved in decision-making. In the context of Monte Carlo simulations, running sensitivity tests can shed light on which parameters exert the greatest influence on the outcome.
Follow these guidelines for effective sensitivity analysis:
- Identify Key Variables: Determine which inputs produce the most significant shifts in outcomes. Focusing on these can help allocate resources to monitor them closely.
- Range Testing: Test a wide array of values within each of the key variables to observe their influence, which could reveal potential vulnerabilities in your financial strategy.
- Iterate and Adjust: It is wise to rerun analyses and refine your model based on findings. Keeping your simulations dynamic allows adaptation to changing financial landscapes.
Regularly Updating Input Data
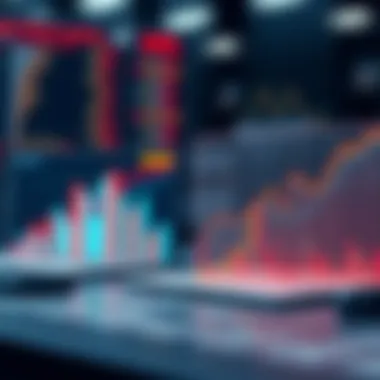
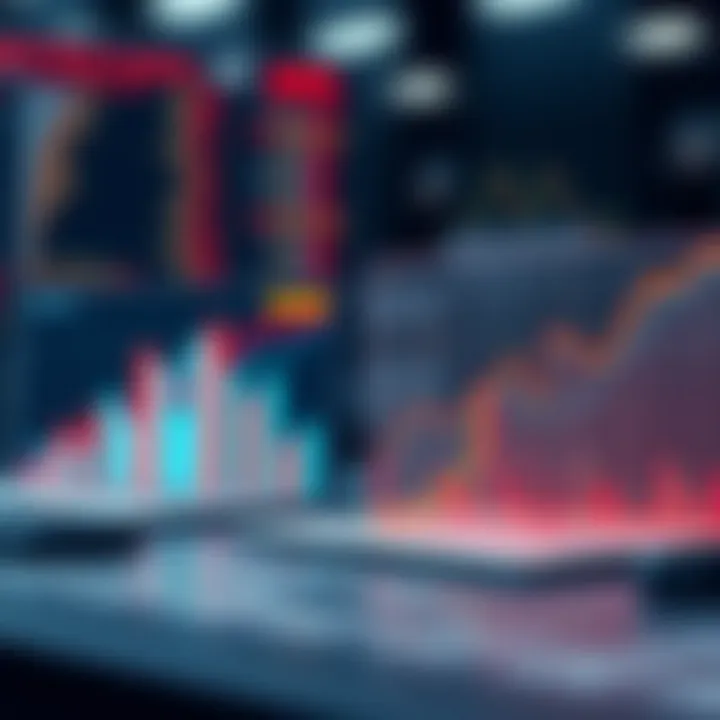
Data is not static; it’s fluid and continually evolves. Regularly refreshing input data is essential to keeping your Monte Carlo simulations relevant and reliable. Using outdated or stale data can distort results and lead to poor financial decisions.
Here are several tactics for maintaining data accuracy:
- Schedule Updates: Set a regular timetable for reviewing and updating input data. This could be quarterly, biannually, or whenever market conditions demand it.
- Integrate Real-Time Data: If possible, leverage real-time data feeds to ensure that market conditions are reflected in your models consistently.
- Source Multiple Data Points: Cross-reference various sources to validate data accuracy and avoid decisions based on incomplete or potentially biased information.
By integrating these best practices, investors and financial analysts can greatly improve the utility of Monte Carlo analysis software in their decision-making processes. Ensuring realistic parameters, sensitive testing of assumptions, and continuously updating inputs lead to informed decisions that minimize risks and maximize returns.
Case Studies: Successful Applications of Monte Carlo Analysis
Monte Carlo analysis serves as a vital tool within the financial sector, providing a sophisticated approach to evaluating risks and uncertainties. Through case studies, we can observe how this analysis software offers concrete benefits, facilitating informed decision-making in various investment avenues. By exploring real-world examples, investors and financial professionals can better understand the practical applications of these simulations.
Investor Portfolio Optimization
Investor portfolio optimization is a central application of Monte Carlo analysis. The investment landscape is filled with unpredictable variables, from market fluctuations to economic shifts. By employing Monte Carlo simulations, investors can model different scenarios and examine how their portfolios might respond to these uncertainties. This approach helps in:
- Diversifying Risk: By understanding potential outcomes across various asset classes, investors learn how to spread risks more effectively.
- Projecting Returns: The simulations provide insights into the expected return rates based on historical data, allowing investors to make well-founded choices about where to allocate funds.
- Assessing Investment Strategies: Investors can evaluate current strategies against various market conditions and tweak them accordingly for optimal performance.
An interesting case involved a hedge fund using Monte Carlo to forecast their multi-asset portfolio. They discovered that a heavy focus on equities was increasing their risk exposure. The simulation prompted them to diversify into bonds and commodities, leading to a more balanced risk-reward profile.
Risk Management in Banking
In the banking sector, risk management is paramount. Monte Carlo analysis shines in this field by allowing banks to simulate various risk factors affecting their portfolios. This method helps institutions navigate:
- Credit Risk: Banks can evaluate potential default risks among borrowers by analyzing historical default rates and economic conditions.
- Market Risk: By modeling the variability in asset prices, institutions gain insights into how market shocks could impact their financial health.
- Liquidity Risk: The software assists in forecasting scenarios where liquidity may dwindle, enabling banks to keep sufficient reserves.
For instance, a prominent bank utilized Monte Carlo analysis to assess the impact of potential economic downturns on their loan portfolio. The outcome of their simulations highlighted vulnerabilities in specific sectors, prompting them to adjust their lending strategies proactively.
Evaluating Real Estate Investments
When it comes to real estate, Monte Carlo analysis becomes indispensable for evaluating investment opportunities. The unpredictable nature of property prices makes this approach an asset for:
- Cash Flow Projections: Investors can assess potential rental incomes by simulating various economic conditions affecting tenant demand.
- Valuation Assessment: Monte Carlo simulations allow investors to analyze property values under differing market scenarios, providing a clearer picture of potential returns.
- Risk Analysis: Investors can identify potential pitfalls, such as location risks and market downturns, thus making informed decisions.
A notable case involved a real estate investment firm that applied Monte Carlo analysis to evaluate a potential acquisition in a fluctuating market. By projecting various price scenarios, they were able to ascertain a reasonable purchase price, ultimately securing a profitable investment driven by data rather than speculation.
Future Perspectives on Monte Carlo Analysis
The future of Monte Carlo analysis in finance is tightly woven into the fabric of emerging technologies and shifting market demands. This section elucidates the significance of anticipating future trends, markets, and regulatory frameworks that shape the landscape of Monte Carlo analysis. Understanding these facets is essential for investors and analysts looking to maintain a competitive edge. By grasping the evolving dynamics, stakeholders can better position themselves to harness the full potential of Monte Carlo simulations in their decision-making frameworks.
Emerging Trends in Financial Analysis
As the financial world continues to evolve, several trends are emerging that are influencing the application of Monte Carlo analysis software.
- Machine Learning Integration: Advanced algorithms and data analytics are enhancing traditional Monte Carlo simulations. These integrations allow for more nuanced risk assessments and better predictive modeling. As machine learning continues to mature, its application in Monte Carlo analysis will only deepen, offering predictive insights that were previously unattainable.
- Increased Data Utilization: The rise of big data means that more information is at analysts' fingertips than ever before. Leveraging this wealth of data allows financial experts to run more robust simulations, draw better conclusions, and ultimately make more informed decisions.
- Real-Time Analysis Capabilities: The demand for more timely insights is growing. Financial analysts are beginning to utilize Monte Carlo simulations in real-time, allowing for instantaneous updates based on fluctuating market conditions. This shift can lead to significantly more agile decision-making processes.
Potential New Markets and Applications
The versatility of Monte Carlo analysis software opens doors to several new markets and applications:
- Cryptocurrency Assessments: As the digital asset space expands, so does the need for robust analytical tools. Monte Carlo analysis can provide vital insights into the volatility and risk profiles of various cryptocurrencies, aiding investors in making educated decisions.
- Sustainable Investment Strategies: The growing focus on environmental, social, and governance (ESG) criteria opens up avenues for Monte Carlo simulations to evaluate the long-term impacts of sustainable investments. By understanding potential risks and rewards, investors can align their portfolios with their values.
- Healthcare Sector Valuations: The complexities surrounding healthcare investments due to regulatory, technological, and demographic factors can be tackled with Monte Carlo analysis. The ability to simulate a range of scenarios can help stakeholders mitigate risks in an ever-changing environment.
Regulatory Considerations
As Monte Carlo analysis finds its way into varying sectors, regulatory concerns naturally arise, necessitating attention and foresight:
"Regulatory landscapes are shifting. Adapting to changes can ensure the continued relevance and application of Monte Carlo methods in finance."
- Compliance Standards: Financial institutions must navigate an increasingly complex web of regulations. Monte Carlo analysis tools need to incorporate compliance features to help users remain within legal frameworks while making investment decisions.
- Data Privacy Regulations: With heightened scrutiny on data privacy, especially in the wake of regulations like GDPR, the way data is collected, used, and stored in Monte Carlo simulations must become a top priority. Compliance with these standards will be crucial to maintaining credibility with clients.
- Transparency Requirements: Regulators are pushing for greater transparency in financial practices. Users of Monte Carlo analysis software must ensure that their methodologies and assumptions are clearly laid out to comply with these regulatory demands while building trust with stakeholders.
End and Recommendations
As we reach the end of our exploration into the complexities of Monte Carlo analysis software, it's essential to underscore the pivotal role it plays in financial decision-making. This conclusion not only recaps the significant details covered but also suggests strategic steps for optimally applying this analytical method in real-world scenarios. The insights gathered will help investors and financial advisors alike in navigating an increasingly intricate investment landscape.
Summarizing Key Insights
Throughout this article, we’ve delved into various dimensions of Monte Carlo simulations. Here are the core takeaways:
- Fundamental Understanding: We examined the foundational principles like random sampling and probability distributions, which are essential for any practitioner using these techniques. By grasping these concepts, users can better appreciate how the software performs calculations and forecasts potential outcomes.
- Application Spectrum: We've highlighted significant applications in areas like portfolio management, risk assessment, and option pricing. Understanding where to deploy Monte Carlo analysis is key to enhancing investment strategies and minimizing potential losses.
- Challenges and Limitations: While powerful, we also discussed the software's limitations, such as its reliance on assumptions and potential computational complexity. This insight is important as it helps users to remain critical and discerning about the results produced by simulations.
- Technological Advancements: Advancements in machine learning and cloud integration are revolutionizing how Monte Carlo methods are employed. It's crucial for users to keep abreast of these changes to fully leverage the capabilities available to them.
In summary, a strong grasp of the tool's dynamics, capable of transforming how financial decisions are approached, is indispensable.
Future Directions for Research and Development
Looking ahead, research and developments will continue to shape the way Monte Carlo analysis functions within financial models. Focus on these areas for future investigations:
- Integration with Big Data: As data sets grow, enhancing Monte Carlo methods to handle larger volumes effectively will increase their accuracy and reliability. This can elevate them from theory to practical, actionable strategies in decision-making.
- Automation and Real-Time Analysis: Streamlining the simulations to run in real-time can empower investors to make swift choices. Incorporating automation into the analysis process can free analysts to focus on strategy, rather than repetitive calculations.
- Collaboration Across Disciplines: Engaging with fields like behavioral finance can help refine modeling techniques that anticipate market reactions better. This interdisciplinary approach might unveil hidden layers of complexity in financial decision-making processes.
These directions may lead to sophisticated tools that could define the new standard in investment analysis.
Encouraging Broader Adoption of Monte Carlo Methods
For Monte Carlo analysis to achieve its full potential, broad adoption is crucial. Here are some steps to consider:
- Educational Initiatives: Financial education programs should incorporate Monte Carlo simulations into standard curricula. Empirical knowledge combined with theoretical understanding can significantly elevate a practitioner's effectiveness in the field.
- User-Friendly Software Design: Simplifying the interfaces of Monte Carlo software can promote greater use. More intuitive systems enable users with less technical expertise to tap into the power of simulations.
- Community Engagement: Building forums and discussion groups for investors and analysts can facilitate knowledge sharing and troubleshoot common issues. Platforms like Reddit or Facebook can be effective spaces for intellectual exchange and community-driven learning.
Ultimately, fostering a culture that embraces advanced data-driven methodologies can help modernize financial practices, making them more efficient and predictive.